In a world where data drives decisions, and speed determines success, businesses face an important question:
How can we use AI to enhance agility without losing our human touch?
The answer lies in RAG (Retrieval-Augmented Generation), a cutting-edge technology that bridges the gap between raw data and actionable insights.
What is RAG (Retrieval-Augmented Generation)?
At its core, RAG combines two powerful elements of artificial intelligence:
1.Retrieval: The ability to pull the most relevant data from vast sources (like internal documents, databases, or even the web).
2.Augmented Generation: Using this data to generate meaningful, context-aware responses or outputs.
Imagine having a supercharged assistant that doesn’t just provide vague recommendations but delivers precise, data-backed solutions tailored to your current needs. That’s RAG in action!
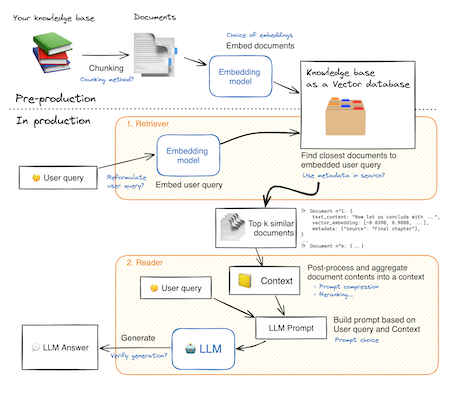
How RAG Can Boost Agility?
Agility is all about responding quickly and effectively to change, and RAG is a perfect ally for that. Here’s how:
1. Faster Decision-Making
Agile teams thrive on informed decisions made in real-time. RAG minimizes the time spent searching for information by delivering the most relevant insights instantly.
Example: A Scrum team needing market trends to prioritize backlog items can access them in seconds through RAG-driven insights.
2. Improved Collaboration
RAG supports transparent and precise communication, ensuring everyone on the team works with the same accurate information.
Example: A product owner can retrieve customer feedback data to align development goals without sifting through emails or outdated spreadsheets.
3. Personalized Coaching for Teams
RAG enables tailored guidance based on team dynamics and project needs, offering AI-powered coaching that adapts in real-time.
Example: A newly-formed Agile team can receive suggestions for retrospectives or sprint improvements based on historical data.
4. Enhanced Risk Management
With RAG, risks can be identified early, and mitigation strategies can be informed by past scenarios or best practices.
Example: Spotting bottlenecks in delivery pipelines based on historical velocity trends and suggesting preemptive actions.
5. Scalability Without Complexity
Traditional scaling frameworks can feel cumbersome. RAG enables simplicity by integrating with tools your team already uses, enhancing—not replacing—them.
Example: Automating team health checks and delivering insights during sprint planning.
RAG in Action: Use Cases for Agility
•Knowledge Management: Automatically summarizing and distributing sprint outcomes to stakeholders.
•Customer Feedback: Analyzing reviews and support tickets to prioritize features.
•Team Dynamics: Identifying patterns in retrospectives to improve collaboration.
•Real-Time Reporting: Providing immediate, insightful updates during daily stand-ups or reviews.
The Future of Agile and RAG
In the AI-driven workplace, RAG doesn’t just complement agility; it redefines it. Agile is no longer limited to processes or frameworks. With RAG, it becomes a living, breathing system that evolves continuously—faster, smarter, and more human-centered.
Contact Us
“Agility isn’t just about doing things faster—it’s about doing the right things, the right way, at the right time.”
Are you ready to embrace RAG and redefine your Agile practices?
Request a free workshop session from artwork@sysart.consulting.
References
Retrieval-Augmented Generation:
•Lewis, Patrick et al. “Retrieval-Augmented Generation for Knowledge-Intensive NLP Tasks.” arXiv preprint arXiv:2005.11401 (2020). Access on arXiv
Practical Applications of RAG
•Hugging Face: Guides and tutorials for building retrieval-augmented generation systems.
Flow image source:
“What is RAG? – Retrieval-Augmented Generation AI Explained”
This article from Amazon Web Services (AWS) offers a clear explanation of RAG, detailing how it enhances large language models by referencing external knowledge bases to generate more accurate and context-aware responses.
What is RAG (Retrieval-Augmented Generation)?
“Retrieval Augmented Generation (RAG) in Azure AI Search”
This Microsoft documentation provides an overview of how RAG architecture augments large language models by integrating information retrieval systems, offering insights into its implementation within Azure AI Search.